With the museum’s Director of External Affairs, Roger Highfield, and Prof Ed Dougherty of Texas A&M, Peter Coveney of University College London has written a critique of the blind use of big data in biology. Here Prof Coveney sums up their paper.
Visit the Our Lives in Data exhibition in the Science Museum’s Wellcome Wing and you will see a section on the revolution in genetics. Today it is cheaper than ever to read a person’s entire genetic code (genome), since a public consortium and private venture unveiled the very first examples back in June 2000, the former having spent billions of dollars on what was hailed as biology’s answer to the moon shot.
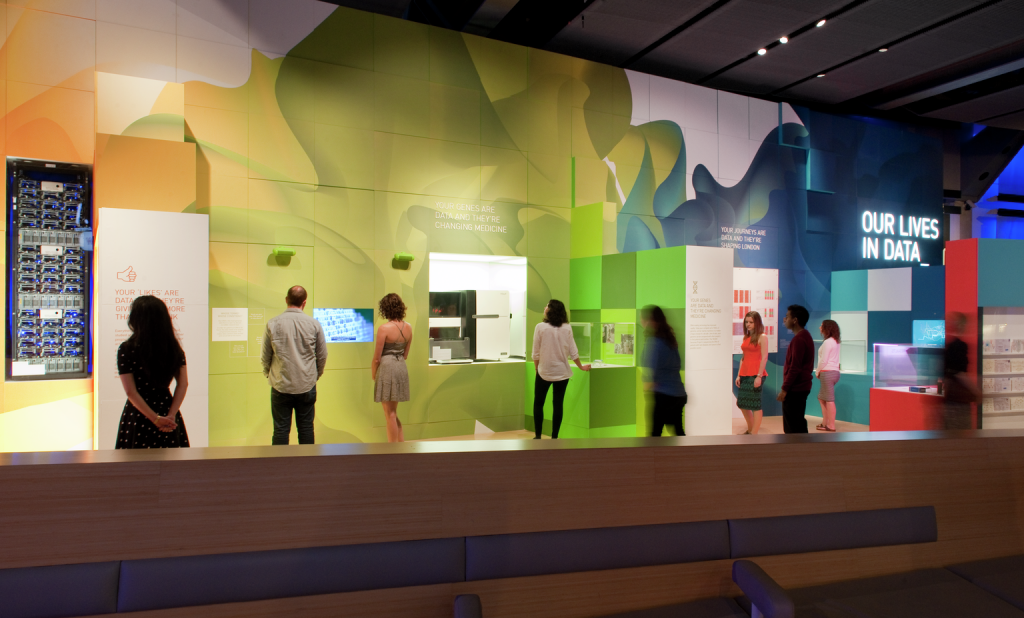
Hundreds of thousands of human genomes have since been read in the quest to develop new tests and treatments and in the UK, Genomics England has a major venture known as the 100,000 genomes project, to create a new genomic medicine service for the NHS to transform the way people are looked after.
However, as one caption in the exhibition tellingly reads, the real challenge now is sorting through all these data. And, as I explain in a paper published today in the Philosophical Transactions of the Royal Society A, biology is too complex to rely on data that have been blindly harvested. We need more theory and understanding if we are to make sense of this tsunami of data and omes – genomes, proteomes and transcriptomes.
Mathematical modelling is relatively primitive in biology compared to physics and for understandable reasons: living things are hugely complicated. Think of the mind-boggling complexity of a cell, organ or brain.
But with the rise of big data – 90% of the data currently in the world was created in the last two years – there is growing confidence that biology will yield its secrets and some have even made the extraordinary claim that that computers can run powerful statistical algorithms to discern patterns where science cannot: we no longer need theory.
With Dr Roger Highfield, one of the museum’s directors, and Prof Ed Dougherty of Texas A&M, we argue in the paper – based on a presentation given earlier this year at a Solvay meeting in Brussels and a meeting in Santa Fe, New Mexico – that this is misleading and, though big biological data will be extremely valuable, biology needs big theory too, if it is to make real progress.
One hint that data are not enough comes from looking at the clinical returns of the human genome project, which have been decidedly underwhelming compared with the ballyhoo and hype that surrounded the unveiling of the first genomes in the White House by teams led by Craig Venter and Francis Collins.
Back then, people would talk about how a person’s genome would allow you to develop ‘personalised medicine’. But because we don’t understand how an individual’s genetic code translates into treatments – not least because we don’t understand enough about the role of epigenetics, the environment and so on – ‘personalised medicine’ has been quietly downgraded to ‘precision medicine’, where we look at how genetically similar people react and then assume that a given person will respond in a similar way.
To make sense of all these data, researchers reach for a type of artificial intelligence primarily built on artificial neural networks. In effect, they say that “based on the people we have seen and treated before, we expect the patient in front of us now to do this”. But no matter their “depth” and sophistication, these neural nets merely fit curves to existing data.
They generally fail in circumstances beyond the range of the data used to train them because tiny changes in the molecular structure of a potential drug can lead to dramatic differences in potency. Blind data dredging is most likely to produce correlations that are spurious rather than meaningful. And they are silent about what mechanisms are at play, and this is the kind of understanding we need to design new kinds of drug.
Because it takes so much data to describe a biological system, one needs to know which data is important for a particular objective. Physicists already understand this. The discovery of the Higgs boson at CERN’s Large Hadron Collider required petabytes of data but relied on theory to guide the search for this new particle. Nor do we predict tomorrow’s weather by averaging historic records of that day’s weather – mathematical models do a much better job with the help of daily data pouring down to Earth from satellites.
Because every person is different, the only way to use a person’s genetic information to predict how an individual will react to a drug is if we have a profound understanding of how the body works, and its physical characteristics, so that we can model the way that each person will absorb and interact with the drug molecule.
This sounds simple but drug companies have been trying this approach for decades and, though successful at helping to guide the search for new treatments, we are still unable to take a person’s genetic makeup and predict, in a matter of hours, which treatment is the most suitable.
My team at University College London has started to show how it should soon be possible to do this with the help of sophisticated modelling, heavyweight computing and smart statistical analysis. We use supercomputers to model how a candidate drug molecule tumbles towards its target in the body and to explore how it interacts, if at all.
You can think of it as trying to figure out how a key (drug) fits into a lock (a target protein such as an enzyme, or one in a disease agent) but the complication is that these are stochastic processes, things which have random components and are simply too complex to be properly understood. To overcome this, in our work we use what is called a form Monte Carlo simulation imposed on the determinism of Newtonian physics where we study how well the key fits into the adjusting lock over myriad random circumstances of speed, vibration orientation and so on.
In the longer term, we are also working on virtual humans, so treatments can be tested on a digital Doppelgänger of a patient as a trial run before treatment. Today a new EU CompBioMed Centre of Excellence will be launched at University College London to help realise this dream.
But we need to do even more: we need to figure out the laws of biology to make sense of all these data.
Our Lives in Data is a free exhibition at the Science Museum until August 2017. Find out more at sciencemuseum.org.uk/data.
Peter Coveney is a professor in Physical Chemistry and Director of the Centre for Computational Science at University College London, Professor Adjunct at Yale University, and leads the #CompBioMed initiative.